Enhancing Agricultural Resilience through Remote Sensing and AI:
Modern agriculture faces significant challenges from climate change, limited water resources, rising production costs, and disruptions like the COVID-19 pandemic. These issues jeopardize the sustainability of food production systems, necessitating innovative solutions to meet the demands of a growing global population. Recent advancements in remote sensing and AI have opened new avenues for improving crop monitoring and management. By integrating these technologies, we can gather and analyze large-scale phenotypic data with unprecedented accuracy, facilitating the development of predictive and prescriptive management tools.
The study shows how remote sensing technologies, such as Unmanned Aircraft Systems (UAS), can be combined with AI to enhance agricultural systems’ resilience and efficiency. UAS provides detailed field-scale data, which can improve farm decision-making and system management when integrated with AI-driven analytics. Despite these advancements, challenges remain in adopting UAS technologies and incorporating them with spaceborne remote sensing data for broader applications. The study explores these challenges and prospects for leveraging these technologies to address national and global agricultural and nutritional difficulties.
Unmanned Aerial Systems (UAS) Revolutionizing Digital Agriculture:
UAS offers a cost-effective, efficient alternative to traditional field sensors, which can be costly and disruptive to agricultural operations. UAS equipped with sensors provide high-resolution, temporal, and spatial data on plant growth, surpassing conventional methods that often miss in-field variations. Recent research highlights the growing interest in UAS for agriculture, with applications ranging from assessing plant phenotypes and water stress to monitoring diseases and estimating yields. While UAS adoption faces data processing and interpretation challenges, their integration with AI promises to enhance agricultural efficiency and productivity.
Integrating Genomics and Phenomics with UAS:
Advanced genomics has enhanced crop breeding by identifying genetic markers linked to complex traits. However, bridging the gap between genomic data and phenotypic expression requires accurate field-level measurements. UAS addresses this need by providing high-resolution, consistent phenotypic data. Studies have shown that UAS can effectively measure traits like plant height and canopy cover, complementing genomic tools and improving breeding accuracy. By integrating UAS with genomic analysis, researchers can uncover detailed genotype-phenotype relationships, enhance marker-assisted breeding, and accelerate the development of superior crop varieties.
Combining Remote Sensing, Simulation Models, and AI in Digital Agriculture:
UAS offers efficient crop phenotyping, but their spatial coverage is limited by battery life and data processing costs. While satellite data is used for broader applications, it lacks the resolution needed for precision agriculture. Integrating high-resolution UAS data with satellite information can enhance large-scale predictions. Advances in ML and crop simulation models provide opportunities to improve accuracy and scalability. By combining these technologies, we can develop robust models for yield estimation and in-season management, bridging gaps between field-scale data and large-scale agricultural applications.
Future Directions in Sustainable Crop Management:
journal journal journal journal journal journal journal journal journal journal journal journal journal journal journal journal journal journal journal journal journal journal journal journal journal journal journal journal journal journal journal journal journal journal journal journal journal journal journal
To advance sustainable crop management, we must enhance resource efficiency in agriculture. Technological progress offers promising solutions for the coming decades, with UAS proving effective in quantifying field-scale phenotypic data and accelerating breeding cycles when integrated with Genomic Wide Association Studies (GWAS). Although still developing, combining UAS with spaceborne remote sensing, AI, and crop simulation models paves the way for large-scale digital agriculture applications. Future efforts should focus on building multidisciplinary teams and establishing standardized data collection and analysis protocols. Ensuring high-quality raw data remains crucial for the success of these advancements.
Check out the Paper. All credit for this research goes to the researchers of this project. Also, don’t forget to follow us on Twitter and join our Telegram Channel and LinkedIn Group. If you like our work, you will love our newsletter..
Don’t Forget to join our 48k+ ML SubReddit
Find Upcoming AI Webinars here
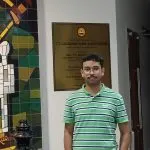
Sana Hassan, a consulting intern at Marktechpost and dual-degree student at IIT Madras, is passionate about applying technology and AI to address real-world challenges. With a keen interest in solving practical problems, he brings a fresh perspective to the intersection of AI and real-life solutions.