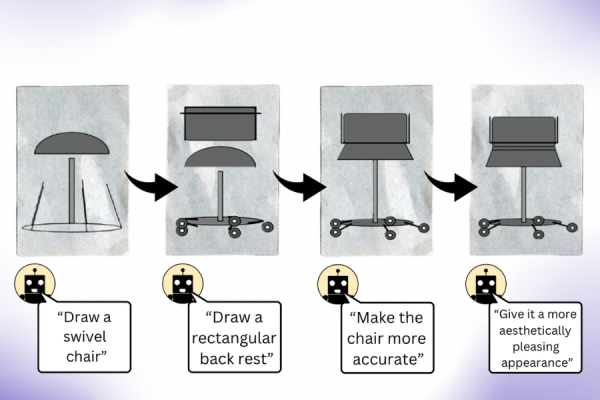
Understanding the visual knowledge of language models | MIT News
You’ve likely heard that a picture is worth a thousand words, but can a large language model (LLM) get the picture if it’s never seen images before? As it turns out, language models that are trained purely on text have a solid understanding of the visual world. They can write image-rendering code to generate…