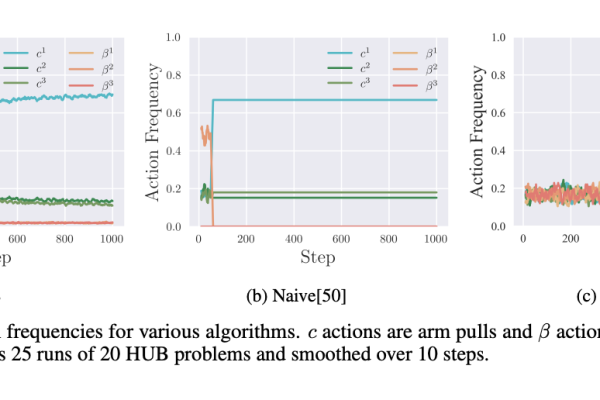
Researchers from UC Berkeley and Stanford Introduce the Hidden Utility Bandit (HUB): An Artificial Intelligence Framework to Model Learning Reward from Multiple Teachers
In Reinforcement learning (RL), effectively integrating human feedback into learning processes has risen to the forefront as a significant challenge. This challenge becomes particularly pronounced in Reward Learning from Human Feedback (RLHF), especially when dealing with multiple teachers. The complexities surrounding the selection of teachers in RLHF systems have led researchers to introduce the…